Artificial Intelligence (AI) has transformed the way we perceive technology, and at the heart of this revolution lies the intricate architecture of AI Neural Networks. These networks, inspired by the human brain, have become the backbone of various AI applications, from image recognition to natural language processing. In this article, we will take a deep dive into the world of AI Neural Networks, unraveling their structure, functioning, and the impact they have on our daily lives.
Introduction
Definition of Artificial Intelligence (AI)
AI refers to the simulation of human intelligence in machines, enabling them to perform tasks that typically require human intelligence. Neural Networks are a subset of AI, mimicking the human brain’s neural structure.
Significance of Neural Networks in Artificial Intelligence (AI)
Neural Networks play a pivotal role in AI, allowing machines to learn from data, recognize patterns, and make decisions. Understanding their intricacies is essential for grasping the potential of AI.
Basics of Neural Networks
Structure and Components
Neural Networks consist of interconnected nodes, or neurons, organized in layers. These layers include the input layer, hidden layers, and output layer. Each connection has a weight, and the network adjusts these weights during the learning process.
Functioning of Neurons
Neurons in a Neural Network process information through activation functions. These functions determine whether a neuron should be activated based on the input it receives, enabling the network to make complex decisions.
Types of Neural Networks
Feedforward Neural Networks
This type of network transmits data in one direction, from the input layer to the output layer. It is commonly used in tasks like image and speech recognition.
Recurrent Neural Networks
In contrast, recurrent networks allow information to be looped back, enabling them to consider previous inputs. This makes them suitable for tasks requiring context, such as language modeling.
Convolutional Neural Networks
Designed for image processing, Convolutional Neural Networks excel in tasks like object recognition. Their architecture involves convolutional layers that extract features from input data.
How Neural Networks Learn
Training Data
Neural Networks learn from labeled training data, adjusting their weights to minimize the difference between predicted and actual outputs.
Activation Functions
Activation functions introduce non-linearities, enabling Neural Networks to learn complex relationships within data.
Backpropagation
During training, errors are propagated backward through the network, adjusting weights to improve performance. This iterative process refines the network’s ability to make accurate predictions.
Applications of Neural Networks
Image Recognition
Neural Networks power image recognition technologies, allowing machines to identify objects and patterns in visual data.
Natural Language Processing
In the realm of language, Neural Networks excel at tasks like sentiment analysis, language translation, and chatbot interactions.
Autonomous Vehicles
The ability to process vast amounts of data in real-time makes Neural Networks crucial for the development of autonomous vehicles.
Advancements in Neural Networks
Deep Learning
Deep Learning involves Neural Networks with multiple hidden layers, enabling them to automatically extract hierarchical features from data.
Generative Adversarial Networks (GANs)
GANs consist of two neural networks—generator and discriminator—competing to generate realistic data. This innovative approach has applications in image and video synthesis.
Challenges and Limitations
Overfitting
Neural Networks may become too specialized in the training data, resulting in poor generalization to new data—known as overfitting.
Lack of Interpretability
The complex nature of Neural Networks raises challenges in interpreting their decision-making processes, posing ethical concerns.
Computational Resources
Training sophisticated Neural Networks demands significant computational power, limiting accessibility for some applications.
Future Trends in Neural Networks
Explainable AI
Addressing the lack of interpretability, Explainable AI aims to make Neural Networks’ decision-making processes more transparent and understandable.
Neuromorphic Computing
Inspired by the human brain’s architecture, Neuromorphic Computing seeks to build hardware that mimics neural networks, potentially improving efficiency and scalability.
Real-world Examples
AlphaGo
The success of AlphaGo, an AI system developed by DeepMind, showcased the power of Neural Networks in strategic decision-making.
Voice Assistants
Voice-activated assistants like Siri and Alexa utilize Neural Networks to understand and respond to natural language commands.
Ethical Considerations
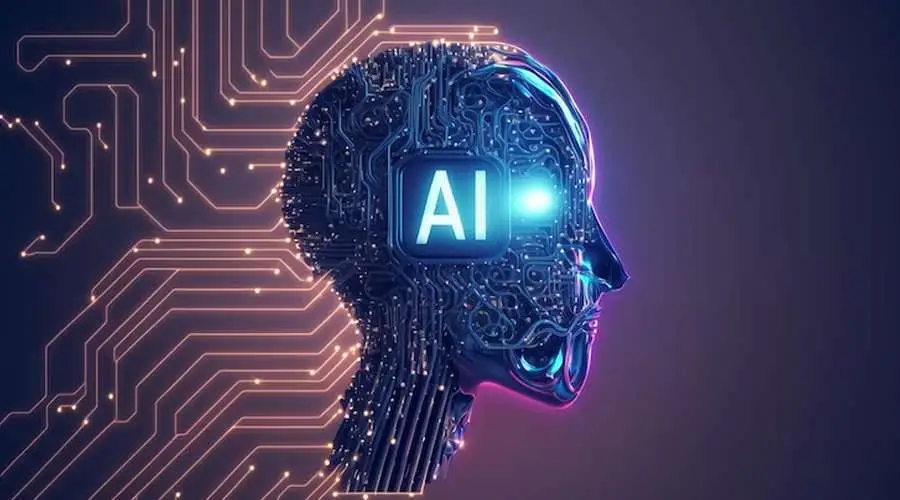
Bias in Artificial Intelligence (AI)
Neural Networks may perpetuate biases present in training data, emphasizing the importance of ethical considerations in AI development.
Privacy Concerns
The extensive use of Neural Networks in processing personal data raises concerns about privacy and data security.
Impact on Industries
Healthcare
Neural Networks contribute to diagnostic accuracy in medical imaging, drug discovery, and personalized medicine.
Finance
In finance, Neural Networks analyze vast datasets to predict market trends, enhance risk management, and automate trading strategies.
How Businesses Can Harness Neural Networks
Implementing AI Strategies
Businesses can leverage Understanding Neural Networks by incorporating AI strategies into their operations, enhancing efficiency and decision-making.
Collaborating with AI Experts
Collaborating with AI experts allows businesses to tailor Neural Network solutions to their specific needs, fostering innovation and competitiveness.
The Role of AI in Society
Job Automation
The widespread use of Neural Networks raises concerns about job automation, prompting a reevaluation of workforce skills and job roles.
Education and Skills Development
As AI becomes more prevalent, there is a growing need for education and skills development to prepare individuals for AI-related roles and challenges.
The Future of Neural Networks
Continuous Innovation
The field of Neural Networks continues to evolve, with ongoing research and innovations driving the development of more advanced and efficient models.
Integration with Other Technologies
Neural Networks are increasingly integrated with other technologies, such as robotics and IoT, expanding their applications across diverse domains.
Conclusion
In conclusion, the journey into the realm of Neural Networks unveils a landscape of innovation and possibilities. As technology advances, so does our understanding of AI, and Neural Networks stand at the forefront of this transformation. From solving complex problems to shaping industries, the impact of Neural Networks is profound and ever-expanding.
FAQs
What is the main purpose of Neural Networks in Artificial Intelligence (AI)?
Neural Networks in AI are designed to mimic the human brain’s ability to learn and make decisions, enabling machines to recognize patterns, process information, and perform complex tasks.
How do Neural Networks learn from data?
Neural Networks learn from labeled training data by adjusting the weights of connections between neurons. This process, known as backpropagation, minimizes the difference between predicted and actual outputs.
What are the challenges associated with Neural Networks?
Challenges include overfitting, lack of interpretability, and the demand for significant computational resources, limiting accessibility for some applications.
How are Neural Networks applied in real-world scenarios?
Neural Networks find applications in image recognition, natural language processing, healthcare diagnostics, financial analysis, and more.
What does the future hold for Understanding Neural Networks?
The future of Understanding Neural Networks involves continuous innovation, integration with other technologies, and addressing ethical considerations, paving the way for a dynamic and impactful future.